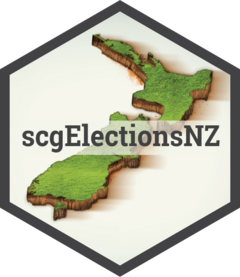
Party and Candidate Votes
Source:vignettes/articles/party-and-candidate-votes.rmd
party-and-candidate-votes.rmd
This article offers a detailed analysis of the party_votes
and candidate_votes
datasets in the scgElectionsNZ
package, shedding light on
the voting dynamics in New Zealand’s elections.
Overview
The party_votes
and candidate_votes
datasets provide comprehensive insights into party preferences and
candidate popularity across electorates. These datasets are pivotal for
understanding how New Zealand’s Mixed-Member Proportional (MMP) system
translates individual and party preferences into electoral outcomes.
Loading the Data
Begin by loading the party_votes
and candidate_votes
datasets to understand their structure and the information they
contain:
Party Votes
Election | Ballot | Electorate | Party | Votes |
---|---|---|---|---|
2023 | Party | Auckland Central | ACT Party | 3301 |
2023 | Party | Auckland Central | Animal Justice Party | 50 |
2023 | Party | Auckland Central | Aotearoa Legalise Cannabis Party | 142 |
2023 | Party | Auckland Central | DemocracyNZ | 32 |
2023 | Party | Auckland Central | Freedoms NZ | 48 |
2023 | Party | Auckland Central | Green Party | 8503 |
2023 | Party | Auckland Central | Informal | 85 |
2023 | Party | Auckland Central | Labour Party | 8028 |
2023 | Party | Auckland Central | Leighton Baker Party | 3 |
2023 | Party | Auckland Central | Maori Party | 563 |
Candidate Votes
# Load data
candidate_df <- scgUtils::get_data("candidate_votes")
# View data
head(candidate_df, 10)
Election | Ballot | Electorate | Party | Votes | Percentage |
---|---|---|---|---|---|
2023 | Candidate | Auckland Central | ACT Party | 1235 | 3.59 |
2023 | Candidate | Auckland Central | Green Party | 16624 | 48.26 |
2023 | Candidate | Auckland Central | Labour Party | 2608 | 7.57 |
2023 | Candidate | Auckland Central | National Party | 12728 | 36.95 |
2023 | Candidate | Auckland Central | NZ First | 0 | 0.00 |
2023 | Candidate | Auckland Central | Maori Party | 0 | 0.00 |
2023 | Candidate | Auckland Central | Other | 1252 | 3.63 |
2023 | Candidate | Banks Peninsula | ACT Party | 2073 | 4.24 |
2023 | Candidate | Banks Peninsula | Green Party | 8325 | 17.03 |
2023 | Candidate | Banks Peninsula | Labour Party | 17464 | 35.72 |
As you can see, the candidate_votes
dataset places all unsuccessful parties into “Other” and does not
include Informal votes.
Combining Party and Candidate Vote Data
Amend Data
To resolve this issue, use the update_names()
function to amend the party names:
# Amend Party names
party_df <- update_names(party_df) # default output = "party"
party_df <- party_df %>%
filter(Party != "Informal") %>% # Remove "Informals"
# Get grouped sum of Votes (i.e., combine all "Other" votes into one):
group_by(Election, Electorate, Ballot, Party) %>%
summarise(Votes = sum(Votes), .groups = 'drop') %>%
ungroup() %>%
# Get grouped percentage and round to 2 decimal places:
group_by(Election, Electorate, Ballot) %>%
mutate(Percentage = Votes / sum(Votes) * 100) %>%
ungroup()
# View amended data
head(party_df, 10)
Election | Electorate | Ballot | Party | Votes | Percentage |
---|---|---|---|---|---|
2023 | Auckland Central | Party | ACT Party | 3301 | 9.33 |
2023 | Auckland Central | Party | Green Party | 8503 | 24.03 |
2023 | Auckland Central | Party | Labour Party | 8028 | 22.69 |
2023 | Auckland Central | Party | Maori Party | 563 | 1.59 |
2023 | Auckland Central | Party | NZ First | 1351 | 3.82 |
2023 | Auckland Central | Party | National Party | 11751 | 33.22 |
2023 | Auckland Central | Party | Other | 1881 | 5.32 |
2023 | Banks Peninsula | Party | ACT Party | 3919 | 7.91 |
2023 | Banks Peninsula | Party | Green Party | 9763 | 19.70 |
2023 | Banks Peninsula | Party | Labour Party | 13200 | 26.63 |
Merge Data
Then, combine both datasets for a unified analysis:
# Combine the two data frames
combined_df <- rbind(party_df, candidate_df)
Reshaping the Data
Reshape the data to understand how party and candidate preferences align or diverge:
combined_df <- combined_df %>%
filter(Election == 2023) %>% # Get the 2023 election only
# NB Ballot is amended to ensure that there are not two "Party" columns when pivoting wider:
mutate(Ballot = ifelse(Ballot == "Party", "Party Vote", "Candidate Vote")) %>%
select(Election, Ballot, Electorate, Party, Percentage) %>% # Remove Votes column
tidyr::pivot_wider(names_from = Ballot, values_from = Percentage) # Pivot wider
# Make NAs = 0
combined_df[is.na(combined_df)] <- 0
# View data
head(combined_df, 10)
Election | Electorate | Party | Party Vote | Candidate Vote |
---|---|---|---|---|
2023 | Auckland Central | ACT Party | 9.33 | 3.59 |
2023 | Auckland Central | Green Party | 24.03 | 48.26 |
2023 | Auckland Central | Labour Party | 22.69 | 7.57 |
2023 | Auckland Central | Maori Party | 1.59 | 0.00 |
2023 | Auckland Central | NZ First | 3.82 | 0.00 |
2023 | Auckland Central | National Party | 33.22 | 36.95 |
2023 | Auckland Central | Other | 5.32 | 3.63 |
2023 | Banks Peninsula | ACT Party | 7.91 | 4.24 |
2023 | Banks Peninsula | Green Party | 19.70 | 17.03 |
2023 | Banks Peninsula | Labour Party | 26.63 | 35.72 |
Visualising Party and Candidate Votes
Create a visualisation comparing Party and Candidate votes to
understand the correlation between these two types of votes, which can
indicate the degree of split
voting by electorate. The colour_pal()
function from the scgUtils
package can be used to assign
the party colours and the theme_scg()
function from the same package can be used to customise the plot’s
appearance:
combined_df %>%
filter(Party != "Other") %>% # Remove "Other"
ggplot(aes(x = `Candidate Vote`, y = `Party Vote`, colour = Party)) +
geom_smooth(method = "lm", se = TRUE, formula = y ~ x, # Add linear regression
colour = scgUtils::colour_pal("French Grey"),
linewidth = 0.5, fill = "#F4F4F5", alpha = 0.5) +
geom_point(na.rm = TRUE) + # Add scatter plot
ggpmisc::stat_poly_eq(colour = scgUtils::colour_pal("Regent Grey")) + # Add R^2
facet_wrap(. ~ Party) + # Facet by Party
scale_colour_manual(values = scgUtils::colour_pal("polNZ")) + # Get party colours
coord_equal(ylim = c(0, 80), xlim = c(0, 80)) + # Make axes equal
labs(title = "2023 Candidate vs Party Vote\nby Electorate and Party", # Add labels
y = "Party Vote (%)\n",
x = "Candidate Vote (%)") +
scgUtils::theme_scg() + # Apply theme
theme(legend.position = "none") # Turn off legend